一般说法是gmm分类器可以用于缺陷检测,mlp不支持缺陷检测。
勇哥还是想试试mlp到底能不能用于缺陷检测。
于是有了下面的实验。
这个实验用到的图片,原来是gmm分类器的一个倒子用到的。
dev_close_window() read_image (Image, 'plastic_mesh/plastic_mesh_01') get_image_size (Image, Width, Height) dev_open_window(0, 0, Width, Height, 'black', WindowHandle) get_system ('example_dir', HalconExamples) gen_rectangle1 (Rectangle, 10, 10, Height / 2 - 11, Width / 2 - 11) * 创建多层感知器,5维特征向量维度,6层神经元的隐藏层,输出2类,softmax分类器(多项式分布),预处理设置为'principal_components',使用主成分分析,特征向量所在的组件数3,随机数初始化种子是42 create_class_mlp (5, 6, 2, 'softmax', 'principal_components', 3, 42, MLPHandle) * The training is based on five images that contain no errors. *生成空区域 gen_empty_region (EmptyRegion) *把矩形区域放入空区域中 concat_obj (Rectangle, EmptyRegion, ObjectsConcat) *抽取5张图片的特征 for J := 1 to 5 by 1 read_image (Image, 'plastic_mesh/plastic_mesh_' + J$'02') *缩小图片,减少运行时间 zoom_image_factor (Image, ImageZoomed, 0.5, 0.5, 'constant') dev_display (ImageZoomed) disp_message (WindowHandle, 'Adding training samples...', 'window', 10, 10, 'black', 'true') *抽取纹理特征,见网格缺陷检测(GMM)博客 gen_texture_image (ImageZoomed, ImageTexture) *把特征和空区域添加到训练句柄中 add_samples_image_class_mlp (ImageTexture, ObjectsConcat, MLPHandle) endfor *设置拒绝采样策略'hyperbox_ring_around_each_class' set_rejection_params_class_mlp (MLPHandle, 'sampling_strategy', 'hyperbox_ring_around_each_class') *设置拒绝采样策略因子3 set_rejection_params_class_mlp (MLPHandle, 'rejection_sample_factor', .3) *开始训练,MaxIterations优化算法的最大迭代次数200, WeightTolerance最优算法在两次迭代的不同权重的阈值是1,两次迭代的平均错误的差值阈值0.01,Error训练数据的平均错误, ErrorLog作为一个优化算法的迭代次数函数在训练数据上的平均错误 train_class_mlp (MLPHandle, 200, 1, 0.01, Error, ErrorLog) for J := 1 to 14 by 1 read_image (Image, 'plastic_mesh/plastic_mesh_' + J$'02') *缩小图片 zoom_image_factor (Image, ImageZoomed, 0.5, 0.5, 'constant') *抽取特征图 gen_texture_image (ImageZoomed, ImageTexture) *裁剪特征图得到需要分类的区域 reduce_domain (ImageTexture, Rectangle, ImageTextureReduced) *分类 classify_image_class_mlp (ImageTextureReduced, ClassRegions, MLPHandle, 0.5) *分成两类区域,正确区域和错误的区域 select_obj (ClassRegions, Correct, 1) select_obj (ClassRegions, Errors, 2) * 错误区域开运算和闭运算,去除细小的区域 opening_circle (Errors, ErrorsOpening, 4.5) closing_circle (ErrorsOpening, ErrorsClosing, 10.5) connection (ErrorsClosing, ErrorsConnected) *筛选面积大于100的区域 select_shape (ErrorsConnected, FinalErrors, 'area', 'and', 100, 1000000) count_obj (FinalErrors, NumErrors) dev_set_color ('red') dev_set_draw ('margin') dev_set_line_width (5) dev_display(ImageZoomed) dev_display (FinalErrors) if (NumErrors > 0) disp_message (WindowHandle, 'Mesh not OK', 'window', 10, 10, 'black', 'true') else disp_message (WindowHandle, 'Mesh OK', 'window', 10, 10, 'black', 'true') endif if (J < 14) disp_continue_message (WindowHandle, 'black', 'true') endif stop () endfor clear_class_mlp (MLPHandle)
本地函数的源码:
texture_laws (Image, ImageEL, 'el', 5, 5) texture_laws (Image, ImageLE, 'le', 5, 5) texture_laws (Image, ImageES, 'es', 1, 5) texture_laws (Image, ImageSE, 'se', 1, 5) texture_laws (Image, ImageEE, 'ee', 2, 5) compose5 (ImageEL, ImageLE, ImageES, ImageSE, ImageEE, ImageLaws) smooth_image (ImageLaws, ImageTexture, 'gauss', 5) return ()
可以看到,我们实现了跟gmm一样的效果。
---------------------
作者:hackpig
来源:www.skcircle.com
版权声明:本文为博主原创文章,转载请附上博文链接!
本文出自勇哥的网站《少有人走的路》wwww.skcircle.com,转载请注明出处!讨论可扫码加群: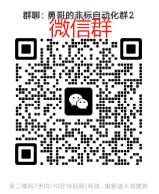
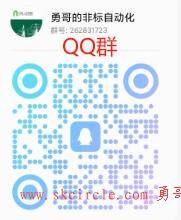
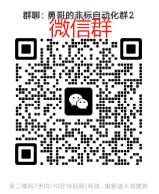
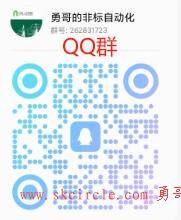