前言:
==========================================================
分类器相对于深度学习来讲是一种古老传统的图片处理技术。halcon中常见的有四类分类器:
MLP(多层神经网络neural Nets)
SVM(支持向量机)
K-NN(K-最邻近)
GMM(高斯混合类型)
分类器的应用领域主要是下面这些:
image segmentation 图像分割
object recognition 对象识别
quality control 质量控制
novelty detection 缺陷检测
optical character recognition(OCR) 光学字符识别
勇哥第一次见到分类器的视觉项目是锂电池的极片缺陷检测,效果还不错。
这两年深度学习火起来后,发现深度学习完成上面所说的领域的应用更容易,效果也更好。
但深度学习对硬件要求太高,你把IPC加装个一百多W的显卡很多时候是不现实的。
如果你用cpu来跑,会发现速度乎快乎慢,cpu全部内核会100%被占用。
分类器相对于深度学习来讲不吃硬件,所以相对来讲算是轻量级的应用。
==========================================================
下面这个示例程序展示了如何使用MLP像素分类器来分割基于纹理的图像,MLP像素分类器直接或间接地与查找表分类器(LUT)一起使用。
为了构造一个用于分类的六通道图像,使用了六个滤波器
LUT的解释
LUT是查找表,在MLP,GMM,SVM等分类器中都有对应的LUT算子。
LUT创建完成后就包含了MLP所有的响应信息,不需要再对图片中每个像素点进行分类,所以能极大的提升速度。
LUT的缺点是,分类效果没有标准分类方式精确。
MLP对应的创建LUT的算子签名如下:
create_class_lut_mlp(::MLPHandle,GenParamNames,GenParamValues:ClassLUTHandle)
除了算子create_class_lut_mlp本身的参数外,先前创建MLP分类器的算子create_class_mlp的几个参数对LUT也很重要。
NumInput,
Preprocessing,
NumComponents
create_class_mlp( : : NumInput, NumHidden, NumOutput, OutputFunction, Preprocessing, NumComponents, RandSeed : MLPHandle)
在NumInput中,定义了必须对图像进行分类的图像通道数。通过使用Preprocessing ,可以将图像通道的数量转换为NumComponents。
NumComponents定义特征向量的长度,分类器classify_class_mlp在内部处理该特征向量 。
由于性能和磁盘空间的原因,LUT被限制为最大3维。
由于它代替了运算符classify_class_mlp,因此 NumComponents必须 <= 3。
如果没有减少图像通道数量的Preprocessing(NumInput = NumComponents),则可以使用classify_class_mlp对可能出现在字节图像中的所有像素值 进行分类。
如果存在减少图像通道数量的Preprocessing(NumInput > NumComponents),则MLP的预处理参数存储在LUT的单独结构中。
为了创建LUT,所有转换后的像素值都使用classify_class_mlp进行分类。
在下面的示例程序中,为了创建LUT,必须把添加样本,和训练MLP的流程走完,然后才可以创建LUT。
create_class_mlp (6, 6, 8, 'softmax', 'principal_components', 3, 42, MLPHandle) add_samples_image_class_mlp (TextureImage, ClassSamples, MLPHandle) …… train_class_mlp (MLPHandle, 200, 1, 0.01, Error, ErrorLog) …… create_class_lut_mlp (MLPHandle, [], [], ClassLUTHandle)
使用LUT的分类(即算子classify_image_class_lut)精度比classify_image_class_mlp的低。
使用“ bit_depth”和“ class_selection”,可以控制分类的准确性。它们通过create_class_lut_mlp算子的GenParamNames,GenParamValues进行设置。
'bit_depth': 像素使用的位数。它控制LUT分类器的存储需求,并受图像的位深限制('bit_depth' <= 8)。 如果LUT的位深度较小('bit_depth' < 8),则多个像素组合的类别将映射到同一LUT条目, 这可能导致分类的准确性降低。 这些群集之一包含 像素组合,其中NumComponents表示LUT的尺寸,该尺寸在create_class_mlp中指定。 例如,对于'bit_depth' = 7, NumComponents = 3,则8个像素组合的类别 映射在同一LUT条目中。 LUT最多需要 存储字节。 例如,对于NumComponents = 3, 'bit_depth' = 8和NumOutput < 16 (在create_class_mlp中指定), LUT需要8 MB的存储空间并进行内部存储优化。 如果NumOutput = 1,则通过使用LUT的完整位深度,LUT仅需要2 MB的存储空间。 如果LUT适合缓存,则classify_image_class_lut中分类的运行时将变得最少。 该默认值是8,典型的值是[ 6,7,8 ]。限制:'bit_depth' > = 1, 'bit_depth' <= 8。 'class_selection': LUT的类别选择方法。可以修改以控制创建LUT分类器所需的准确性和运行时间。 如果LUT的位深度最大,则将忽略“ class_selection”中的值,因此“ bit_depth” = 8成立。 如果LUT的位深度较小('bit_depth' < 8),则多个像素组合的类别将映射到同一LUT条目。 这些群集之一包含 像素组合,其中NumComponents表示LUT的尺寸,该尺寸在create_class_mlp中指定。 通过选择 'class_selection' = 'best',群集中最常出现的类存储在LUT中。 对于“ class_selection”=“ fast”,仅对簇的一个像素,即,具有最小值的像素(逐分量)进行分类。 返回的类存储在LUT中。在这种情况下,后续分类的准确性可能会降低。 另一方面,可以减少创建LUT所需的运行时间,这与LUT所需的最大存储空间成正比,该存储空间由定义。 该缺省值是“快”,可能的值为[ “快”,“最好”。 'rejection_threshold': 拒绝MLP的不确定分类点的阈值。 该参数表示分类返回的概率度量的阈值(请参阅classify_class_mlp和 valuate_class_mlp)。 概率低于“ rejection_threshold”的所有像素 均未分配给任何类别。的默认值是0.5。 限制:'rejection_threshold' > = 0, 'rejection_threshold' <= 1。
演示程序运行结果解释
首先设置8个类的采样框。
训练完成后,再创建LUT。
create_class_lut_mlp (MLPHandle, [], [], ClassLUTHandle)
创建LUT需要一点时间,但是后面进行分类时会极大的提高速度。
接下来对比使用LUT的分类和不使用LUT的分类,两种情况下的时间对比。
使用LUT分类用:
classify_image_class_lut (TextureImage, ClassRegionsLUT, ClassLUTHandle)
普通分类(不用LUT)用:
classify_image_class_mlp (TextureImage, ClassRegions, MLPHandle, 0.5)
从结果中以看到,基LUT的分类速度是标准分类方式的2.5倍。
请注意,例程中也提到了: 虽然LUT速度快了,但是分类结果还是标准分类最准确。
dev_update_off () dev_close_window () dev_open_window (0, 0, 512, 512, 'black', WindowHandleL) dev_set_draw ('margin') dev_set_line_width (3) dev_set_colored (12) set_display_font (WindowHandleL, 14, 'mono', 'true', 'false') read_image (Image, 'combine') dev_display (Image) * * 使用6个texture_laws过滤器 texture_laws (Image, ImageTexture1, 'ee', 5, 7) texture_laws (Image, ImageTexture2, 'ss', 2, 7) texture_laws (Image, ImageTexture3, 'rr', 0, 7) texture_laws (Image, ImageTexture4, 'ww', 0, 7) texture_laws (Image, ImageTexture5, 'le', 7, 7) texture_laws (Image, ImageTexture6, 'el', 7, 7) * * 计算纹理过滤器输出的局部平均值 MaskWidth := 41 mean_image (ImageTexture1, ImageMean1, MaskWidth, MaskWidth) mean_image (ImageTexture2, ImageMean2, MaskWidth, MaskWidth) mean_image (ImageTexture3, ImageMean3, MaskWidth, MaskWidth) mean_image (ImageTexture4, ImageMean4, MaskWidth, MaskWidth) mean_image (ImageTexture5, ImageMean5, MaskWidth, MaskWidth) mean_image (ImageTexture6, ImageMean6, MaskWidth, MaskWidth) compose6 (ImageMean1, ImageMean2, ImageMean3, ImageMean4, ImageMean5, ImageMean6, TextureImage) * * 为八个类创建训练区域 gen_empty_obj (ClassSamples) R1 := 10 dR := 255 C1 := 80 dC := 125 W := 10 H := 225 for Row := R1 to R1 + dR by dR for Col := C1 to C1 + 3 * dC by dC gen_rectangle1 (ClassSample, Row, Col, Row + H, Col + W) concat_obj (ClassSamples, ClassSample, ClassSamples) endfor endfor dev_display (ClassSamples) disp_message (WindowHandleL, 'ROIs for the training data', 'window', 12, 12, 'black', 'true') disp_continue_message (WindowHandleL, 'black', 'true') stop () * * 创建MLP分类器,添加样本,并对其进行训练 create_class_mlp (6, 6, 8, 'softmax', 'principal_components', 3, 42, MLPHandle) add_samples_image_class_mlp (TextureImage, ClassSamples, MLPHandle) dev_display (Image) dev_display (ClassSamples) Message := 'Training MLP classifier ...' disp_message (WindowHandleL, Message, 'window', 12, 12, 'black', 'true') train_class_mlp (MLPHandle, 200, 1, 0.01, Error, ErrorLog) Message := 'Training MLP classifier ... ready.' disp_message (WindowHandleL, Message, 'window', 12, 12, 'black', 'true') * * 创建MLP基于LUT的分类器 Message[1] := 'Creating MLP-based LUT classifier ...' disp_message (WindowHandleL, Message, 'window', 12, 12, 'black', 'true') count_seconds (t1) create_class_lut_mlp (MLPHandle, [], [], ClassLUTHandle) count_seconds (t2) TimeLUTCreate := t2 - t1 Message[1] := 'Creating MLP-based LUT classifier ... ready.' Message[2] := 'Time to create the LUT: ' + TimeLUTCreate$'.2f' + ' s' disp_message (WindowHandleL, Message, 'window', 12, 12, 'black', 'true') disp_continue_message (WindowHandleL, 'black', 'true') stop () * * 用基于MLP的LUT分类器对图像进行分割(分类). count_seconds (t1) for Index := 1 to 100 by 1 classify_image_class_lut (TextureImage, ClassRegionsLUT, ClassLUTHandle) endfor count_seconds (t2) TimeLUT := (t2 - t1) / 100 dev_set_draw ('fill') dev_display (ClassRegionsLUT) disp_message (WindowHandleL, 'Result of the LUT classifier\nTime to classify: ' + TimeLUT$'.4f' + ' s', 'window', 12, 12, 'black', 'true') * * 用MLP分类器对图像进行分割(分类)。 * 注:直接使用MLP分类器的结果更准确,因为基于MLP的LUT分类器需要对减少的信道进行离散化 dev_open_window (0, 512 + 5, 512, 512, 'black', WindowHandleR) dev_set_draw ('fill') dev_set_line_width (3) dev_set_colored (12) dev_display (Image) set_display_font (WindowHandleR, 14, 'mono', 'true', 'false') count_seconds (t1) classify_image_class_mlp (TextureImage, ClassRegions, MLPHandle, 0.5) count_seconds (t2) TimeMLP := t2 - t1 dev_display (ClassRegions) disp_message (WindowHandleR, 'Result of the MLP classifier\nTime to classify: ' + TimeMLP$'.4f' + ' s', 'window', 12, 12, 'black', 'true') TimeSpeedUpMLP := (TimeMLP / TimeLUT - 1.0) * 100.0 disp_message (WindowHandleL, 'Speed-Up: ' + TimeSpeedUpMLP$'.2f' + ' %', 'image', 60, 12, 'dark green', 'true')
---------------------
作者:hackpig
来源:www.skcircle.com
版权声明:本文为博主原创文章,转载请附上博文链接!
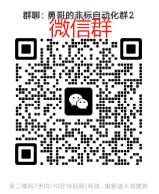
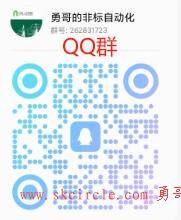