转载自:https://blog.csdn.net/akadiao/article/details/80081842
分类器简介
机器学习在Helcon中的一个重要应用就是用于图像分类任务。Halcon中常用的分类器有GMM(高斯混合模型)、Neural Nets(神经网络)、SVM(支持向量机)等。一般应付常见的分类问题,这些就足够了。
使用方法
一般使用过程:
创建分类器(create_class_…)
获取各个类别的特征向量
将各个类别训练样本的特征向量添加到分类器中(add_sample_class_…)
训练(train_class_…)
获取待分类图像的特征向量
通过分类器计算特征向量的类(classify_class_…)
清除分类器(clear_class_…)
当然,若希望在训练结束后保存训练模型,然后每次使用时通过读取训练模型来实现分类的话,则需要添加模型的保存(write_class_…)与读取(read_class_)。
应用示例
分类对象0为:
以MLP分类器为例:
图像和代码
read_image(Image1, 'Big/大帽正常2017-2-16_16_5_520.bmp') Classes := ['Big','Small'] *获取特征向量 gen_features (Image1, FeatureVector0) *创建一个多层感知器 create_class_mlp (|FeatureVector0|, 15, |Classes|, 'softmax', 'normalization', 10, 42, Handle) *训练分类0list_files ('Big', ['files','follow_links'], ImageFiles) tuple_regexp_select (ImageFiles, ['\\.(tif|tiff|gif|bmp|jpg|jpeg|jp2|png|pcx|pgm|ppm|pbm|xwd|ima|hobj)$','ignore_case'], ImageFiles)for Index := 0 to |ImageFiles|-1 by 1 read_image (Image, ImageFiles[Index]) threshold (Image, region1, 1, 255) reduce_domain(Image, region1, ImageReduced1) crop_domain(ImageReduced1, ImagePart1) gen_features (ImagePart1, FeatureVector) *把一个训练样本添加到一个多层感知器的训练数据中 add_sample_class_mlp (Handle, FeatureVector, 0) endfor *训练分类1list_files ('Small', ['files','follow_links'], ImageFiles) tuple_regexp_select (ImageFiles, ['\\.(tif|tiff|gif|bmp|jpg|jpeg|jp2|png|pcx|pgm|ppm|pbm|xwd|ima|hobj)$','ignore_case'], ImageFiles)for Index := 0 to |ImageFiles| - 1 by 1 read_image (Image, ImageFiles[Index]) * threshold(Image, Region, 1, 255) reduce_domain(Image, Region, ImageReduced) crop_domain(ImageReduced, ImagePart) gen_features (ImagePart, FeatureVector1) *把一个训练样本添加到一个多层感知器的训练数据中 add_sample_class_mlp (Handle, FeatureVector1, 1) endfor *训练一个多层感知器 train_class_mlp (Handle, 200, 1, 0.0001, Error, ErrorLog) *测试分类效果 confidence:=[] list_files ('test', ['files','follow_links'], ImageFiles) tuple_regexp_select (ImageFiles, ['\\.(tif|tiff|gif|bmp|jpg|jpeg|jp2|png|pcx|pgm|ppm|pbm|xwd|ima|hobj)$','ignore_case'], ImageFiles)for Index := 0 to |ImageFiles| - 1 by 1 read_image (Image, ImageFiles[Index]) * threshold(Image, Region1, 1, 255) reduce_domain(Image, Region1, ImageReduced2) crop_domain(ImageReduced2, ImagePart2) gen_features (ImagePart2, FeatureVector2) *通过多层感知器计算一个特征向量的类 classify_class_mlp (Handle, FeatureVector2, 2, FoundClassIDs, Confidence) confidence:=[confidence,Confidence] if (FoundClassIDs[0]=0) dev_display(Image) disp_message (3600, ImageFiles[Index], 'window', 0, 0, 'black', 'true') disp_message (3600, 'Result=大帽', 'window', 20, 0, 'black', 'true') stop() else dev_display(Image) disp_message (3600, ImageFiles[Index], 'window', 0, 0, 'black', 'true') disp_message (3600, 'Result=小帽', 'window', 20, 0, 'black', 'true') stop() endif endfor stop() *清除多层感知器 clear_class_mlp (Handle)
其中函数gen_features(Image,FeatureVector)为:
即将图像的水平与竖直方向的灰度投影作为图像的特征向量。
FeatureVector := [] gray_projections(Image, Image, 'simple', HorProjection, VertProjection) FeatureVector := [HorProjection,VertProjection] return ()
分类效果如图:
常用特征
area_center_gray(Regions, Image: Area, Row, Column)计算一个灰度值图像的区域面积和重心。
cooc_feature_image计算共生矩阵并导出其灰度值特征。
cooc_feature_matrix从一个共生矩阵计算灰度值特征。
elliptic_axis_gray(Regions, Image,Ra, Rb, Phi)在一个灰度值图像中计算一个区域的方位和主轴。
entropy_gray(Regions, Image,Entropy, Anisotropy)确定一个图像的熵和各向异性。
fit_surface_first_order通过一个一阶表面(平面)计算灰度值力矩和近似值。
fuzzy_entropy确定区域的模糊熵。
fuzzy_perimeter计算一个区域的模糊周长。
gen_cooc_matrix (Regions, Image : Matrix : LdGray, Direction : ) 计算图像中区域的共生矩阵。
gray_histo计算灰度值分布。
gray_histo_abs(Regions, Image : Quantization:AbsoluteHisto)计算灰度值分布。
gray_projections计算水平和垂直灰度值投影。
histo_2dim计算两通道灰度值图像的直方图。
intensity(Image, Image, Mean, Deviation)计算灰度值的平均值和偏差。
min_max_gray计算区域内的最大和最小灰度值。
moments_gray_plane用平面计算灰度值矩和近似值。
plane_deviation计算灰度值与近似图像平面的偏差。
select_gray选择基于灰度值特征的区域。
shape_histo_all用极限值确定特征的一个直方图。
shape_histo_point(Region, Image: Feature, Row, Column : AbsoluteHisto, RelativeHisto)用极限值确定特征的一个直方图。
connect_and_holes(Regions : NumConnected, NumHoles)连接组件和孔的数量。
gray_features()计算一组区域的灰度值特征。
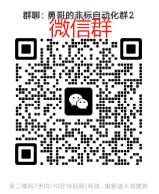
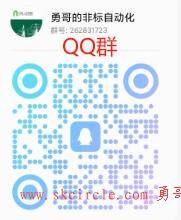