前言:
==========================================================
分类器相对于深度学习来讲是一种古老传统的图片处理技术。halcon中常见的有四类分类器:
MLP(多层神经网络neural Nets)
SVM(支持向量机)
K-NN(K-最邻近)
GMM(高斯混合类型)
分类器的应用领域主要是下面这些:
image segmentation 图像分割
object recognition 对象识别
quality control 质量控制
novelty detection 缺陷检测
optical character recognition(OCR) 光学字符识别
勇哥第一次见到分类器的视觉项目是锂电池的极片缺陷检测,效果还不错。
这两年深度学习火起来后,发现深度学习完成上面所说的领域的应用更容易,效果也更好。
但深度学习对硬件要求太高,你把IPC加装个一百多W的显卡很多时候是不现实的。
如果你用cpu来跑,会发现速度乎快乎慢,cpu全部内核会100%被占用。
分类器相对于深度学习来讲不吃硬件,所以相对来讲算是轻量级的应用。
==========================================================
童鞋你是不是在为分类特征的选择头大呢?
你是不是以分类特征多多益善,加一堆进去,感觉总有派上用处的特征是吧?
其实,halcon有算子是可以自动帮助你挑选最适合的特征的。
勇哥其实也是今天才知道居然有这种神奇的功能的。
我们来分析一下源码,看看这个神奇功能是怎么工作的。
(1) 检查可用的特征与特征组
下面两个库函数,一个查询可用的特征组名字。
一个用来取特征组所属的全部特征的名字。
*检查可用的特征与特征组 query_feature_group_names (AvailableGroupNames) query_feature_names_by_group (AvailableGroupNames, AvailableFeatureNames, AvailableCorrespondingGroups)
可用的特征组名字列表。
其中“rot_invar”,"scale_invar" 不是知道是什么鬼。
“rot_invar”组下面的特征有:
['area', 'ra', 'rb', 'roundness', 'num_sides', 'num_connected', 'num_holes', 'area_holes', 'max_diameter', 'outer_radius', 'inner_radius', 'circularity', 'compactness', 'convexity', 'rectangularity', 'anisometry', 'bulkiness', 'struct_factor', 'dist_mean', 'dist_deviation', 'euler_number', 'rect2_len1', 'rect2_len2', 'contlength', 'porosity', 'gray_area', 'gray_ra', 'gray_rb', 'gray_min', 'gray_max', 'gray_range', 'gray_mean', 'gray_deviation', 'gray_plane_deviation', 'gray_anisotropy', 'gray_entropy', 'edge_density', 'edge_density_histogram', 'edge_density_pyramid_2', 'edge_density_pyramid_3', 'edge_density_pyramid_4', 'edge_density_histogram_pyramid_2', 'edge_density_histogram_pyramid_3', 'edge_density_histogram_pyramid_4', 'polar_gray_proj', 'polar_grad_proj', 'polar_grad_x_proj', 'polar_grad_y_proj', 'polar_gray_proj_histo']
“scale_invar”
['phi', 'roundness', 'num_sides', 'num_connected', 'num_holes', 'orientation', 'circularity', 'compactness', 'convexity', 'rectangularity', 'anisometry', 'bulkiness', 'struct_factor', 'euler_number', 'rect2_phi', 'porosity', 'gray_phi', 'gray_min', 'gray_max', 'gray_range', 'gray_mean', 'gray_deviation', 'gray_plane_deviation', 'gray_anisotropy', 'gray_entropy', 'gray_hor_proj', 'gray_vert_proj', 'gray_hor_proj_histo', 'gray_vert_proj_histo', 'edge_density', 'edge_density_histogram', 'edge_density_pyramid_2', 'edge_density_pyramid_3', 'edge_density_pyramid_4', 'edge_density_histogram_pyramid_2', 'edge_density_histogram_pyramid_3', 'edge_density_histogram_pyramid_4', 'polar_gray_proj', 'polar_grad_proj', 'polar_grad_x_proj', 'polar_grad_y_proj', 'polar_gray_proj_histo']
"color"
['cielab_mean', 'cielab_dev', 'hls_mean', 'hls_dev', 'rgb_mean', 'rgb_dev']
"custom"
'custom_feature_numlines'
"gray"
['gray_area', 'gray_ra', 'gray_rb', 'gray_phi', 'gray_min', 'gray_max', 'gray_range', 'gray_mean', 'gray_deviation', 'gray_plane_deviation', 'gray_anisotropy', 'gray_entropy', 'gray_hor_proj', 'gray_vert_proj', 'gray_hor_proj_histo', 'gray_vert_proj_histo', 'grad_dir_histo', 'edge_density', 'edge_density_histogram', 'edge_density_pyramid_2', 'edge_density_pyramid_3', 'edge_density_pyramid_4', 'edge_density_histogram_pyramid_2', 'edge_density_histogram_pyramid_3', 'edge_density_histogram_pyramid_4', 'cooc', 'cooc_pyramid_2', 'cooc_pyramid_3', 'cooc_pyramid_4', 'polar_gray_proj', 'polar_grad_proj', 'polar_grad_x_proj', 'polar_grad_y_proj', 'polar_gray_proj_histo']
"region"
['area', 'width', 'height', 'ra', 'rb', 'phi', 'roundness', 'num_sides', 'num_connected', 'num_holes', 'area_holes', 'max_diameter', 'orientation', 'outer_radius', 'inner_radius', 'inner_width', 'inner_height', 'circularity', 'compactness', 'convexity', 'rectangularity', 'anisometry', 'bulkiness', 'struct_factor', 'dist_mean', 'dist_deviation', 'euler_number', 'rect2_phi', 'rect2_len1', 'rect2_len2', 'contlength', 'porosity']
"texture"
['gray_mean', 'gray_deviation', 'gray_plane_deviation', 'gray_anisotropy', 'gray_entropy', 'gray_hor_proj', 'gray_vert_proj', 'gray_hor_proj_histo', 'gray_vert_proj_histo', 'grad_dir_histo', 'edge_density', 'edge_density_histogram', 'edge_density_pyramid_2', 'edge_density_pyramid_3', 'edge_density_pyramid_4', 'edge_density_histogram_pyramid_2', 'edge_density_histogram_pyramid_3', 'edge_density_histogram_pyramid_4', 'cooc', 'cooc_pyramid_2', 'cooc_pyramid_3', 'cooc_pyramid_4']
下面是全部特征项目的名字列表。也就是上面罗列的一大堆合在一起了。
(2)使用calculate_feature_set库函数生成颜色和区域特征列表
使用者还是需要按经验挑选几个用得着的特征组,后面会让halcon自动从特征组里挑选出适合的特征。
FeatureGroups := ['region','color'] get_feature_names (FeatureGroups, FeatureNames) get_feature_lengths (FeatureNames, FeatureLengths)
(3)创建并准备分类器训练数据结构
create_class_train_data (sum(FeatureLengths), ClassTrainDataHandle) set_feature_lengths_class_train_data (ClassTrainDataHandle, FeatureLengths, FeatureNames)
(4)训练10张样图
for I := 1 to 10 by 1 read_image (Image, 'color/pills_class_' + I$'.2d') segment_pills (Image, Pills) …… *计算所有分段药丸的特征并将其存储在训练数据结构中 calculate_features (Pills, Image, FeatureNames, Features) add_sample_class_train_data (ClassTrainDataHandle, 'feature_column', Features, I - 1) * …… endfor
['region','color'] 这两个特征组,它们共有38个特征:
['area', 'width', 'height', 'ra', 'rb', 'phi', 'roundness', 'num_sides', 'num_connected', 'num_holes', 'area_holes', 'max_diameter', 'orientation', 'outer_radius', 'inner_radius', 'inner_width', 'inner_height', 'circularity', 'compactness', 'convexity', 'rectangularity', 'anisometry', 'bulkiness', 'struct_factor', 'dist_mean', 'dist_deviation', 'euler_number', 'rect2_phi', 'rect2_len1', 'rect2_len2', 'contlength', 'porosity', 'cielab_mean', 'cielab_dev', 'hls_mean', 'hls_dev', 'rgb_mean', 'rgb_dev']
这38个特征,通过
calculate_features (Pills, Image, FeatureNames, Features)
生成的Features有350个值。
然后用算子add_sample_class_train_data添加样本数据Features到分类器训练数据结构中去。
add_sample_class_train_data (ClassTrainDataHandle, 'feature_column', Features, I - 1)
因此到此步,添加的样本数据都是“全部”生吞,没有经过halcon挑选的。
(5)从训练数据中自动选择合适的特征
select_feature_set_svm (ClassTrainDataHandle, 'greedy', [], [], SVMHandle, SelectedFeatures, Score)
select_feature_set_svm http://www.skcircle.com/?id=1639
经过这个择优算子处理后,对分类最有意义的特征仅为: ['rgb_mean', 'area']
(6)利用自动选择特征的分类器对试验图像中的药丸进行分类
验证图3张,每张图的药丸为NPills个。
for I := 1 to 3 by 1 read_image (Image, 'color/pills_test_' + I$'.2d') segment_pills (Image, Pills) * 对于所有药丸,计算所选特征 * 使用calculate_feature_set库中的calculate_features过程并对其进行分类。 PillsIDs := [] count_obj (Pills, NPills) for P := 1 to NPills by 1 select_obj (Pills, PillSelected, P) calculate_features (PillSelected, Image, SelectedFeatures, Features) classify_class_svm (SVMHandle, real(Features), 1, Class) …… endfor …… endfor endfor
源码:
*这个例子展示了如何使用calculate_feature_set过程库和自动特征选择来使用支持向量机分类器 *对不同的药丸类型进行分类。 *首先,在一些训练图像中对药丸进行分割。 *然后,计算每个药丸的颜色color和区域region特征列表,并存储在分类器训练数据结构中。 *最后,利用算子select_feature_set_svm自动选择最佳特征,最后将得到的分类器应用于多幅测试图像。 dev_close_window () dev_update_off () read_image (Image, 'color/pills_class_01') dev_open_window_fit_image (Image, 0, 0, -1, -1, WindowHandle) dev_set_draw ('margin') dev_set_line_width (2) set_display_font (WindowHandle, 16, 'mono', 'true', 'false') dev_set_colored (12) * PillNames := ['big_round_red','round_green','small_round_red','yellow_trans','brown','brown_green'] PillNames := [PillNames,'brown_grain','purple','turquese','pink'] PillColors := ['#D08080','#ADC691','#FFB0A1','#D5C398','#B59C87','#BCB3B8','#B7ACA1','#908E99','#97B9BC','#C0ABA9'] * *(1)检查可用的特征与特征组 query_feature_group_names (AvailableGroupNames) query_feature_names_by_group (AvailableGroupNames, AvailableFeatureNames, AvailableCorrespondingGroups) * *(2)使用calculate_feature_set库函数生成颜色和区域特征列表 FeatureGroups := ['region','color'] get_feature_names (FeatureGroups, FeatureNames) get_feature_lengths (FeatureNames, FeatureLengths) * *(3)创建并准备分类器训练数据结构 create_class_train_data (sum(FeatureLengths), ClassTrainDataHandle) set_feature_lengths_class_train_data (ClassTrainDataHandle, FeatureLengths, FeatureNames) (4)训练10张样图 for I := 1 to 10 by 1 *分割药丸 read_image (Image, 'color/pills_class_' + I$'.2d') segment_pills (Image, Pills) dev_display (Image) dev_set_color ('white') dev_display (Pills) disp_message (WindowHandle, 'Collecting ' + PillNames[I - 1] + ' samples', 'window', 12, 12, 'black', 'true') * *计算所有分段药丸的特征并将其存储在训练数据结构中 count_obj (Pills, Number) calculate_features (Pills, Image, FeatureNames, Features) add_sample_class_train_data (ClassTrainDataHandle, 'feature_column', Features, I - 1) * *可视化药丸的处理信息 dev_set_color (PillColors[I - 1]) dev_display (Pills) GroupList := sum('\'' + FeatureGroups + '\', ') tuple_str_first_n (GroupList, strlen(GroupList) - 3, GroupList) Message := 'Calculate ' + |FeatureNames| + ' features from following feature groups:' disp_message (WindowHandle, [Message,GroupList], 'window', 40, 12, 'black', 'true') disp_continue_message (WindowHandle, 'black', 'true') stop () endfor * *(5)从训练数据中自动选择合适的特征 *Selecting optimal features 选择最佳特征 disp_message (WindowHandle, 'Selecting optimal features...', 'window', 90, 12, 'black', 'true') select_feature_set_svm (ClassTrainDataHandle, 'greedy', [], [], SVMHandle, SelectedFeatures, Score) disp_message (WindowHandle, ['Selected:',SelectedFeatures], 'window', 120, 12, 'black', 'true') disp_continue_message (WindowHandle, 'black', 'true') stop () * *(6)利用自动选择特征的分类器对试验图像中的药丸进行分类 dev_set_line_width (4) dev_set_colored (12) for I := 1 to 3 by 1 read_image (Image, 'color/pills_test_' + I$'.2d') dev_display (Image) segment_pills (Image, Pills) * 对于所有药丸,计算所选特征 * 使用calculate_feature_set库中的calculate_features过程并对其进行分类。 PillsIDs := [] count_obj (Pills, NPills) for P := 1 to NPills by 1 select_obj (Pills, PillSelected, P) calculate_features (PillSelected, Image, SelectedFeatures, Features) classify_class_svm (SVMHandle, real(Features), 1, Class) * Display results PillsIDs := [PillsIDs,Class] dev_set_color (PillColors[Class]) dev_display (PillSelected) area_center (PillSelected, Area, Row, Column) disp_message (WindowHandle, Class + 1, 'image', Row, Column - 10, 'black', 'true') endfor disp_message (WindowHandle, 'Classify image ' + I + ' of 3 using following features:', 'window', 12, 12, 'black', 'true') disp_message (WindowHandle, SelectedFeatures, 'window', 40, 12, 'black', 'true') if (I < 3) disp_continue_message (WindowHandle, 'black', 'true') stop () endif endfor
---------------------
作者:hackpig
来源:www.skcircle.com
版权声明:本文为博主原创文章,转载请附上博文链接!
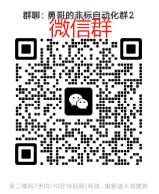
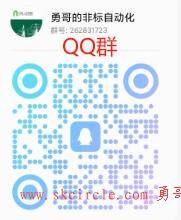